The reproducibility movement in science has sought to increase our confidence in scientific knowledge by having research teams disseminate their data, instruments, and code so that other researchers can reproduce their work. Unfortunately, all approaches to reproducible research to date suffer from the same fundamental flaw: they seek to reproduce the results of previous research while making no effort to reproduce the research process that led to those results. We propose a new method of Exceedingly Reproducible Research (ERR) to close this gap. This blog post will introduce scientists to the error of their ways, and to the ERR of ours.
Even if a replication appears to have succeeded in producing tables and figures that appear identical to those in the original, they differ in that they are providing answers to different questions. An example from our own work illustrates the point.
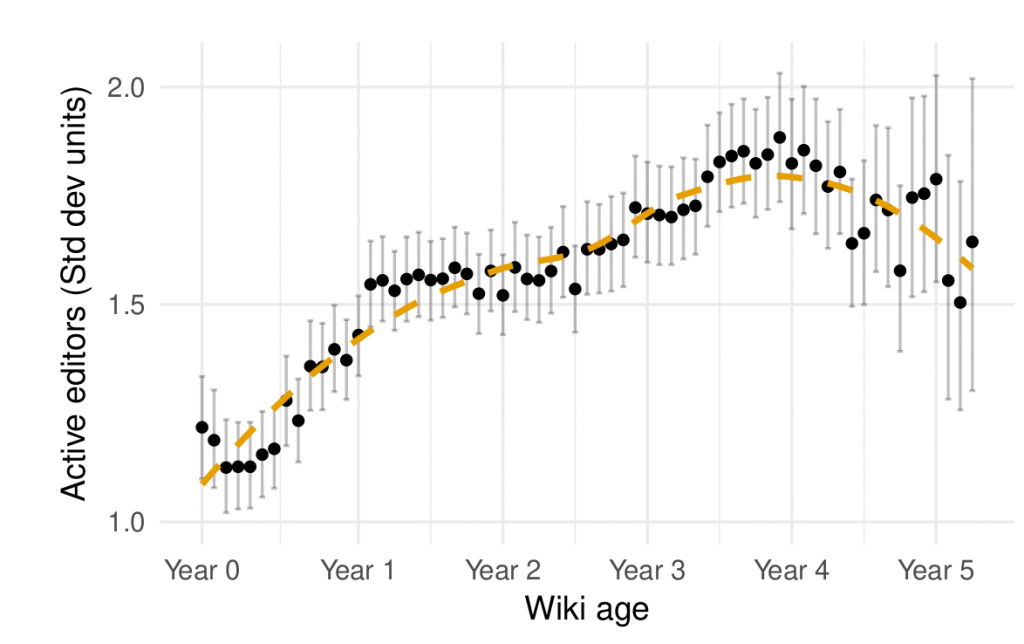
Figure 1 above shows the average number of contributors (in standardized units) to a series of large wikis drawn from Wikia. It was created to show the life-cycles of large online communities and published in a paper last year.
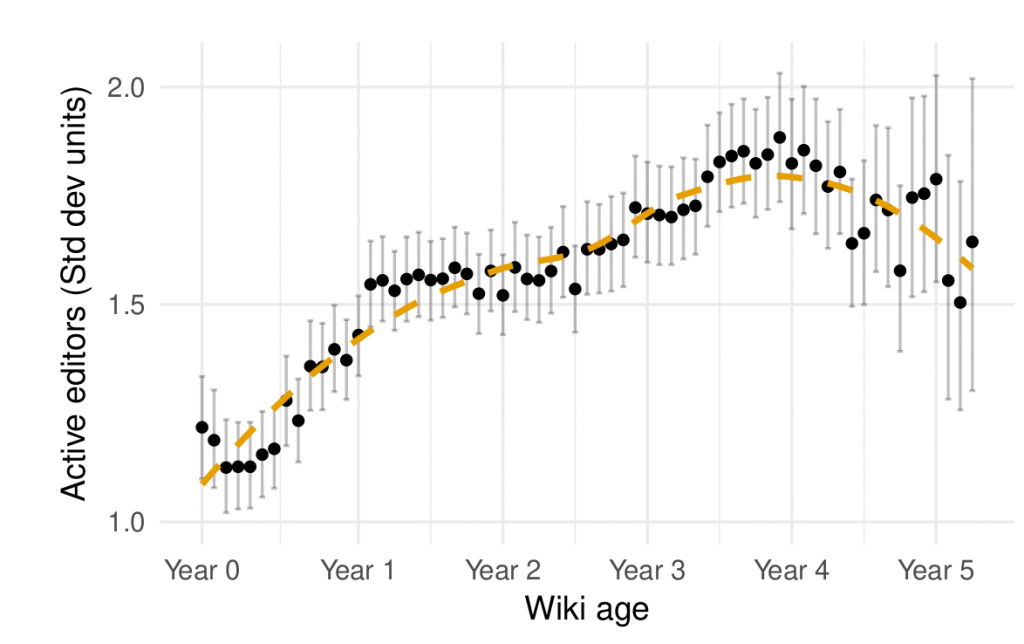
Figure 2: Replication of Figure 1 from TeBlunthuis, Shaw, and Hill (2018)
Results from a replication are shown in Figure 2. As you can see, the plots have much in common. However, deeper inspection reveals that the similarity is entirely superficial. Although the dots and lines fall in the same places on the graphs, they fall there for entirely different reasons.

Figure 1 reflects a lengthy exploration and refinement of a (mostly) original idea and told us something we did not know. Figure 2 merely tells us that the replication was “successful.” They look similar and may confuse a reader into thinking that they reflect the same thing. But they are as different as night as day. We are like Pierre Menard who reproduced two chapters of Don Quixote word-for-word through his own experiences: the image appears similar but the meaning is completely changed. In that we made no attempt to reproduce the research process, our attempt at replication was doomed before it began.
How Can We Do Better?
Scientific research is not made by code and data, it is made by people. In order to replicate a piece of work, one should reproduce all parts of the research. One must retrace another’s steps, as it were, through the garden of forking paths.
In ERR, researchers must conceive of the idea, design the research project, collect the data, write the code, and interpret the results. ERR involves carrying out every relevant aspect of the research process again, from start to finish. What counts as relevant? Because nobody has attempted ERR before, we cannot know for sure. However, we are reasonably confident that successful ERR will involve taking the same courses as the original scientists, reading the same books and articles, having the same conversations at conferences, conducting the same lab meetings, recruiting the same research subjects, and making the same mistakes.
There are many things that might affect a study indirectly and that, as a result, must also be carried out again. For example, it seems likely that a researcher attempting to ERR must read the same novels, eat the same food, fall prey to the same illnesses, live in the same homes, date and marry the same people, and so on. To ERR, one must have enough information to become the researchers as they engage in the research process from start to finish.
It seems likely that anyone attempting to ERR will be at a major disadvantage when they know that previous research exists. It seems possible that ERR can only be conducted by researchers who never realize that they are engaged in the process of replication at all. By reading this proposal and learning about ERR, it may be difficult to ever carry it out successfully.
Despite these many challenges, ERR has important advantages over traditional approaches to reproducibility. Because they will all be reproduced along the way, ERR requires no replication datasets or code. Of course, to verify that one is “in ERR” will require access to extensive intermediary products. Researchers wanting to support ERR in their own work should provide extensive intermediary products from every stage of the process. Toward that end, the Community Data Science Collective has started creating videos of our lab meetings in the form of PDF flipbooks well suited to deposition in our university’s institutional archives. A single frame is shown in Figure 3. We have released our video_to_pdf tool under a free license which you can use to convert your own MP4 videos to PDF.

With ERR, reproduction results in work that is as original as the original work. Only by reproducing the original so fully, so totally, and in such rigorous detail will true scientific validation become possible. We do not so much seek stand on the shoulders of giants, but rather to inhabit the body of the giant. If to reproduce is human; to ERR is divine.