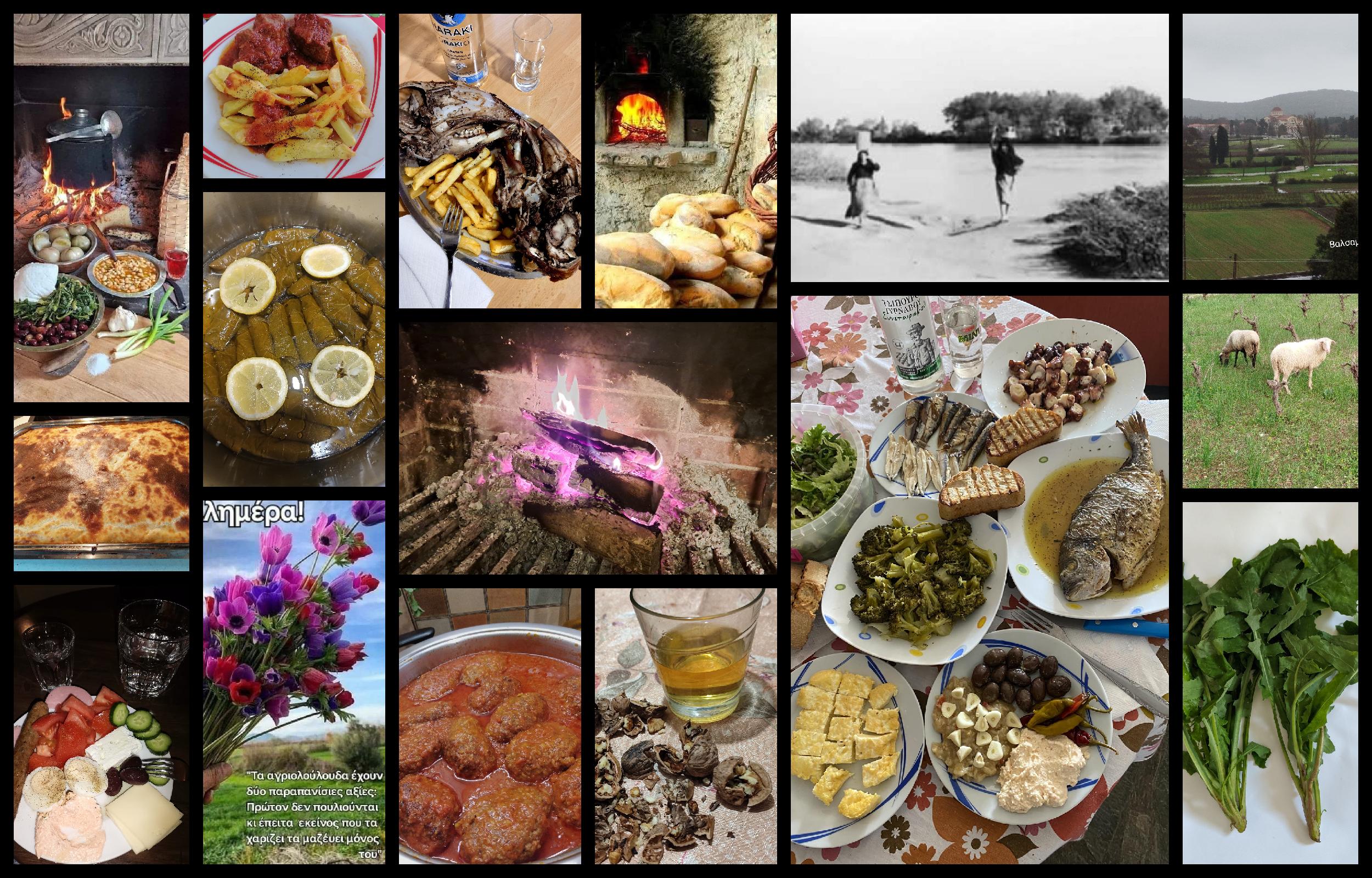
A question that’s been bugging me for the past months is how a digital medium can amplify remnants of traditional pre-modern life.
I’m only a recent addition to the Collective and, despite thinking a lot about digitality and how it affects social processes, I’ve only marginally been part of online communities myself.
However, living back and forth, in a perpetual state of limbo, between Greece and the US for the past two and a half years pursuing graduate school, I’ve inevitably become more active online.
That is when I noticed all these Facebook groups popping up in my feed (yes, I am one of those people who find comfort in the platform’s slower mechanics and less engaging feed).
Groups about life in one’s Greek village, groups about homemade traditional delicacies (as grotesque as lamb intestines and brains), groups about tsipouro (the Greek eau de vie, rakı, grappa, moonshine, …).
Those groups seem to be composed by active middle aged members, who barely know how to use the medium in socially acceptable ways devised by my generation (one of the first one’s in Greece to go online), yet spent hours posting facts about how to make cheese or definitions for words no more used, commenting on each other’s drinking habits, discussing about the “proper” way to make moussaka or arguing about the “proper” meze (small dishes, appetizers, tapas, antipasti, …) for tsipouro. On top of that, youngsters invade them as bystanders, looking, smiling, laughing, making fun of the surreal discussions, uppercase comments, text-to-speech mistakes; chaos.
I’m afraid that these groups are part of the general Zeitgeist in the country: dissapointment and longing for long-lost glory, calmness, or simplicity. It is evident in parts of its cultural production. Books about 19th century Greece, movies and TV series about times of old. A remaining obsession with ancient times. Music referring to the country’s folk traditions.
Relatedly and perhaps subconsciously associated, I recently found myself in a concert by the promising Themos Skandamis. Skandamis produces neo-folk (might I say avantgardish [?] songs) which he writes himself. During his concert, comedic elements imitating the local Cretan accent entered his performance. By the end, as a farewell he beautifully sang a capella a traditional song I’ve never heard before.
It’s lyrics follow (original sourced from here, and freely translated by myself with the benevolent help and suggestions of GPT-4):
Για δες περβό… για δες περβόλιν όμορφο, για δες κατάκρυα βρύση το περιβόλι μας για δες κατάκρυα βρύση το περβό… τ’ όριο περβόλι μας τ’ όμορφο. Κι όσα δέντρα κι όσα δέντρα ‘πεμψεν ο Θιος, μέσα είναι φυτεμένα το περιβόλι μας μέσα είναι φυτεμένα το περβό… τ’ όριο περβόλι μας τ’ όμορφο. Κι όσα πουλιά κι όσα πουλιά πετούμενα, μέσα είναι φωλεμένά το περιβόλι μας μέσα είναι φωλεμένα το περβό… τ’ όριο περβόλι μας τ’ όμορφο. Μέσα σε ‘κεί… μέσα σε ‘κείνα (ν)τα πουλιά, εβρέθη ένα παγώνι το παγωνάκι μας εβρέθη ένα παγώνι το παγώ… τ’ όριο παγώνι μας τ’ όμορφο. Και χτίζει τη και χτίζει τη φωλίτσα του, σε μιας μηλιάς κλωνάρί το παγωνάκι μας σε μιας μηλιάς κλωνάρι το παγώ… τ’ όριο παγώνι μας τ’ όμορφο. | Behold the garden… behold our garden’s beauty, behold the cool spring, our garden behold the cool spring, our gard… our garden’s beauty. And all the trees, all the trees God has sent, are planted in, our garden are planted in, our gard… our garden’s beauty. And all the bird and all the birds that roam the skies, have nested in, our garden have nested in, our gard… our garden’s beauty. Among those birds… among those birds, a peacock found, our little peacock, a peacock found, the pea… our peacock’s beauty. And it builds its… and it builds its nest, on the apple tree’s branch, our peacock on the apple tree’s branch, the pea… our peacock’s beauty. |
I kept wondering, what does it mean, and most importantly why a traditional folk song would mark the end of the concert.
And then it strike me, there is this clear metaphor and parallelism between the peacock and digitality. The peacock appears out of context, yet builds its nest in the garden. Digital media invade our analog lives and impose themselves; they become addictively habitual and naturalized.
What is interesting though here is the reversal of roles. The digital sphere, now established, turned into a garden, leaves room for trad life to invade post-modernity; whether it is a cause or an effect of the world’s turmoil remains to be seen.
On the other hand, perhaps I’m thinking too much.