It’s Ph.D. application season and the Community Data Science Collective is recruiting! As always, we are looking for talented people to join our research group. Applying to one of the Ph.D. programs that the CDSC faculty members are affiliated with is a great way to do that.
This post provides a very brief run-down on the CDSC, the different universities and Ph.D. programs we’re affiliated with, and what we’re looking for when we review Ph.D. applications. It’s close to the deadline for some of our programs, but we hope this post will still be useful to prospective applicants now and in the future.
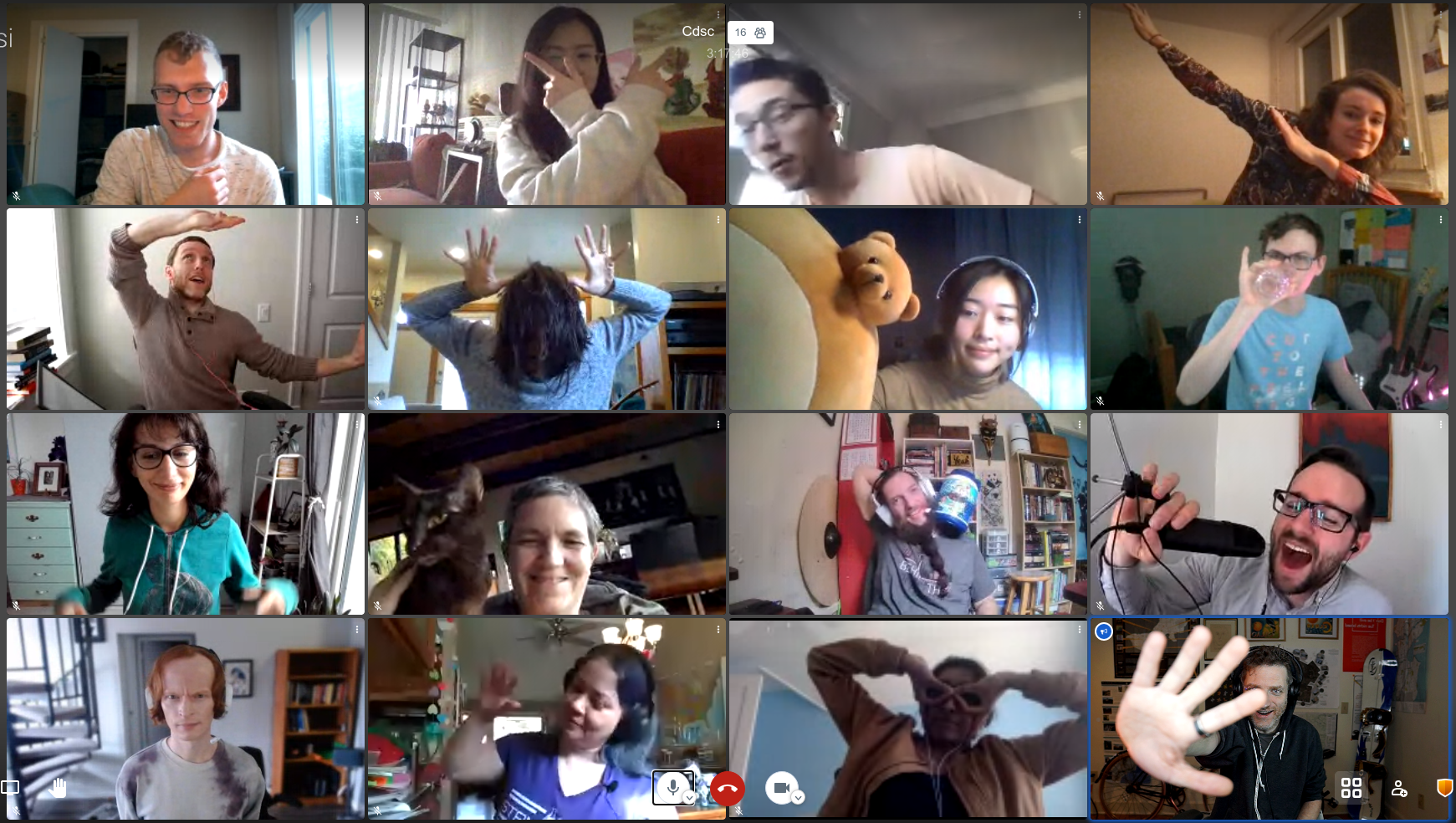
What is the Community Data Science Collective?
The Community Data Science Collective (or CDSC) is a joint research group of (mostly) quantitative social scientists and designers pursuing research about the organization of online communities, peer production, online communities, and learning and collaboration in social computing systems. We are based at Northwestern University, the University of Washington, Carleton College, the University of North Carolina, Chapel Hill, Purdue University, and a few other places. You can read more about us and our work on our research group blog and on the collective’s website/wiki.
What are these different Ph.D. programs? Why would I choose one over the other?
This year the group includes four faculty principal investigators (PIs) who are actively recruiting PhD students: Aaron Shaw (Northwestern University), Benjamin Mako Hill and Sayamindu Dasgupta (University of Washington in Seattle), and Jeremy Foote (Purdue University). Each of these PIs advise Ph.D. students in Ph.D. programs at their respective universities. Our programs are each described below.
Although we often work together on research and serve as co-advisors to students in each others’ projects, each faculty person has specific areas of expertise and interests. The reasons you might choose to apply to one Ph.D. program or to work with a specific faculty member could include factors like your previous training, career goals, and the alignment of your specific research interests with our respective skills.
At the same time, a great thing about the CDSC is that we all collaborate and regularly co-advise students across our respective campuses, so the choice to apply to or attend one program does not prevent you from accessing the expertise of our whole group. But please keep in mind that our different Ph.D. programs have different application deadlines, requirements, and procedures!
Who is actively recruiting this year?
Given the disruptions and uncertainties associated with the COVID19 pandemic, the faculty PIs are more constrained in terms of whether and how they can accept new students this year. If you are interested in applying to any of the programs, we strongly encourage you to reach out the specific faculty in that program before submitting an application.
Ph.D. Advisors
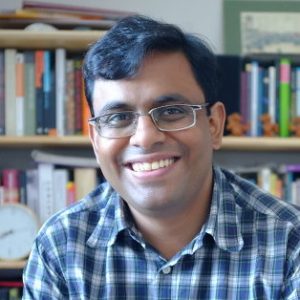
Although he is currently at the University of North Carolina, Sayamindu Dasgupta will starting this year as an Assistant Professor in the Department of Human-Centered Design and Engineering at the University of Washington. Sayamindu’s research focus includes data science education for children and informal learning online—this work involves both system building and empirical studies.
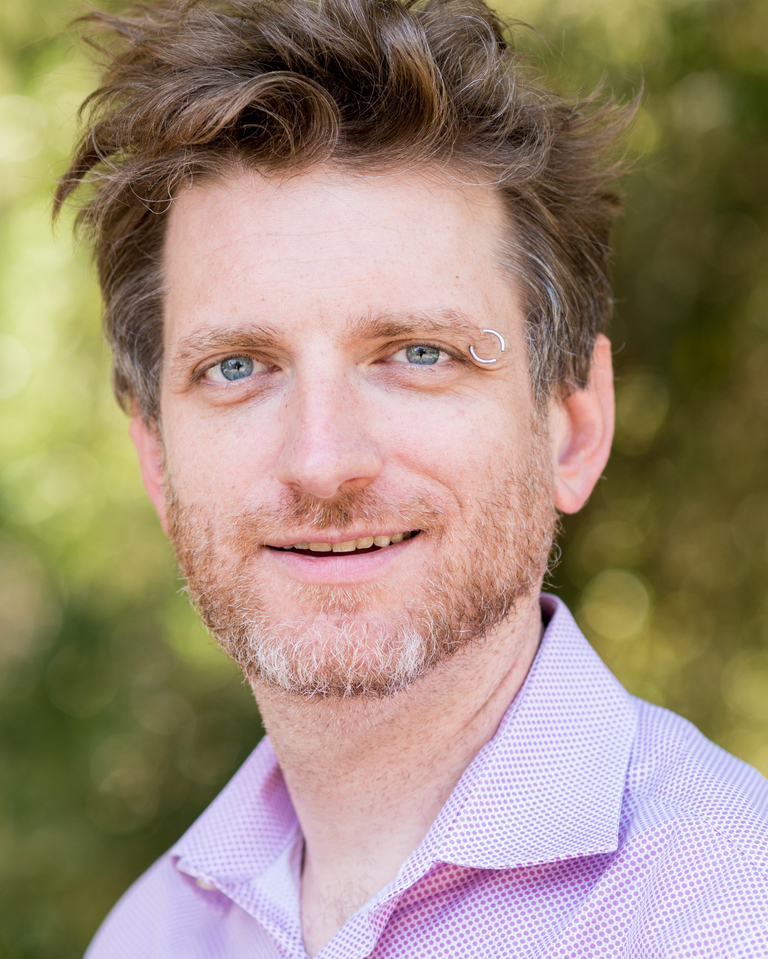
Benjamin Mako Hill is an Assistant Professor of Communication at the University of Washington. He is also an Adjunct Assistant Professor at UW’s Department of Human-Centered Design and Engineering (HCDE), Computer Science and Engineering (CSE) and Information School. Although many of Mako’s students are in the Department of Communication, he has also advised students in all three other departments—although he typically has more limited ability to admit students into those programs. Mako’s research focuses on population-level studies of peer production projects, computational social science, efforts to democratize data science, and informal learning. Mako has also put together a webpage for prospective graduate students with some useful links and information..
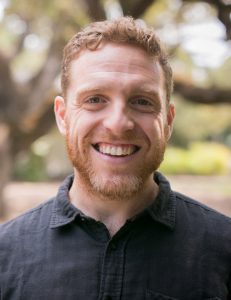
Aaron Shaw is an Associate Professor in the Department of Communication Studies at Northwestern. In terms of Ph.D. programs, Aaron’s primary affiliations are with the Media, Technology and Society (MTS) and the Technology and Social Behavior (TSB) Ph.D. programs. Aaron also has a courtesy appointment in the Sociology Department at Northwestern, but he has not directly supervised any Ph.D. advisees in that department (yet). Aaron’s current research projects focus on comparative analysis of the organization of peer production communities and social computing projects, participation inequalities in online communities, and empirical research methods.
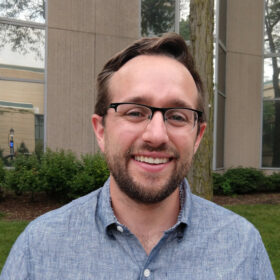
Jeremy Foote is an Assistant Professor at the Brian Lamb School of Communication at Purdue University. He is affiliated with the Organizational Communication and Media, Technology, and Society programs. Jeremy’s current research focuses on how individuals decide when and in what ways to contribute to online communities, how communities change the people who participate in them, and how both of those processes can help us to understand which things become popular and influential. Most of his research is done using data science methods and agent-based simulations.
What do you look for in Ph.D. applicants?
There’s no easy or singular answer to this. In general, we look for curious, intelligent people driven to develop original research projects that advance scientific and practical understanding of topics that intersect with any of our collective research interests.
To get an idea of the interests and experiences present in the group, read our respective bios and CVs (follow the links above to our personal websites). Specific skills that we and our students tend to use on a regular basis include experience consuming and producing social science and/or social computing (human-computer interaction) research; applied statistics and statistical computing, various empirical research methods, social theory and cultural studies, and more.
Formal qualifications that speak to similar skills and show up in your resume, transcripts, or work history are great, but we are much more interested in your capacity to learn, think, write, analyze, and/or code effectively than in your credentials, test scores, grades, or previous affiliations. It’s graduate school and we do not expect you to show up knowing how to do all the things already.
Intellectual creativity, persistence, and a willingness to acquire new skills and problem-solve matter a lot. We think doctoral education is less about executing a task that someone else hands you and more about learning how to identify a new, important problem; develop an appropriate approach to solving it; and explain all of the above and why it matters so that other people can learn from you in the future. Evidence that you can or at least want to do these things is critical. Indications that you can also play well with others and would make a generous, friendly colleague are really important too.
All of this is to say, we do not have any one trait or skill set we look for in prospective students. We strive to be inclusive along every possible dimension. Each person who has joined our group has contributed unique skills and experiences as well as their own personal interests. We want our future students and colleagues to do the same.
Now what?
Still not sure whether or how your interests might fit with the group? Still have questions? Still reading and just don’t want to stop? Follow the links above for more information. Feel free to send at least one of us an email. We are happy to try to answer your questions and always eager to chat.