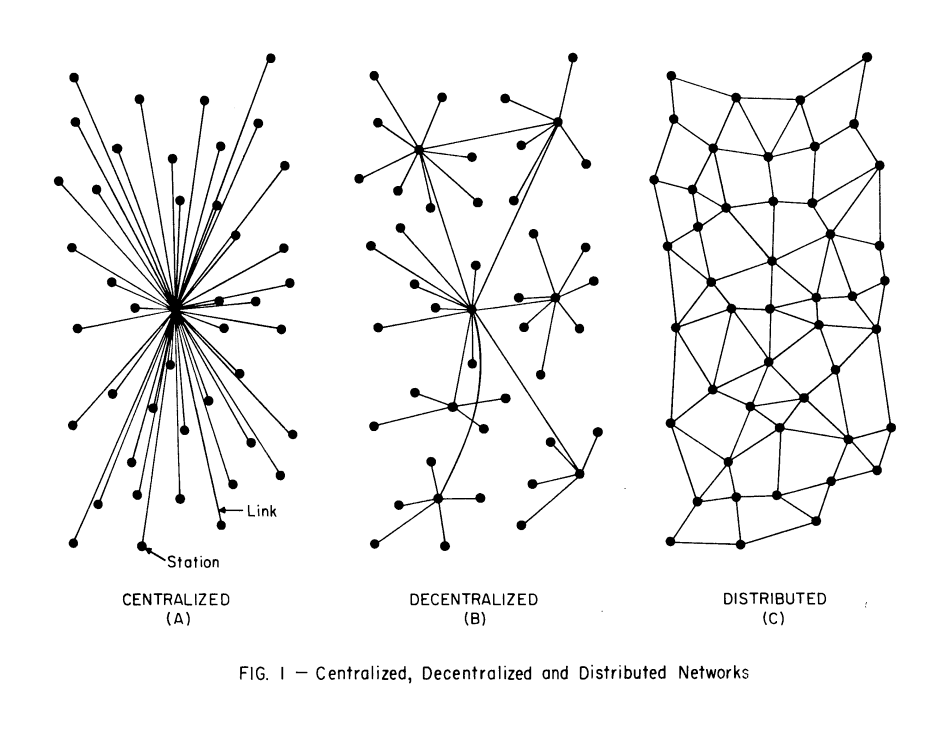
Last Spring, I hosted a “thought leader dialogue” on decentralizing social media through the Northwestern Center for Human-Computer Interaction and Design (HCI+D). It was fantastic and I highly recommend you check out the video.
Afterwards, two of the eponymous “thought-leaders” who headlined that session, Christine Lemmer-Webber and Bryan Newbold followed up with each other in a series of blogposts about decentralization, Bluesky, and the Fediverse.
- How decentralized is Bluesky really? by Christine Lemmer-Webber.
- Reply on Bluesky and decentralization by Bryan Newbold.
- Re: Re: Bluesky and decentralization by Christine Lemmer-Webber
I recently re-read these while preparing for a guest lecture in an undergraduate class here at NU. Fair warning: Despite the subtext of a debate about systems mainly used for micro-blogging, the posts are very long. That said, if you care about these topics (or are maybe just curious to learn more), all three are pure gold.
It helps to understand a bit about exactly who Christine and Bryan are, or more specifically, why they have among the very most informed and interesting perspectives on the topic. Christine helped to create the ActivityPub protocol and standard that is at the heart of the Fediverse (Mastodon, Lemmy, Pixelfed, PeerTube). She currently leads the Spritely Institute pursuing the design and implementation of the next generation of decentralized communication tech. Bryan is the protocol engineer at Bluesky and, as such, one of the leaders building out the AT Protocol, which powers Bluesky and a whole other small-but-seemingly-growing ecosystem of applications.
The crux of their conversation revolves around the competing visions for the future of the social web being pursued within the respective ActivityPub and AT Protocol “universes.” The posts underscore some of the more profound differences between the two, in particular the questions around “shared heap” vs. “message passing” architectures, the relative degree of (de)centralization each system affords (this is where the diagram originally created by Paul Baran and reproduced at the top of this post comes in), and (more implicitly) the theories of change motivating the design and implementation choices prioritized in their respective work.
It’s worth nothing that both Bryan and Christine are very careful and intentional to call out the overlaps between ActivityPub and AT Proto as well. I also appreciate how respectful and thoughtful they each are given that there are some substantive and high stakes areas of disagreement being addressed in their conversation.
If you read the posts (and I hope you do!) and want to share your responses, please do so in the comments, via email, Toot, Skeet, or sundry other means. There are several of us in the lab pursuing work in these areas and I’m eager to understand perspectives on the topic.