A Virtual Thought Leader Dialogue on May 23, from 4 – 5:15 p.m. CST. Register here to join.
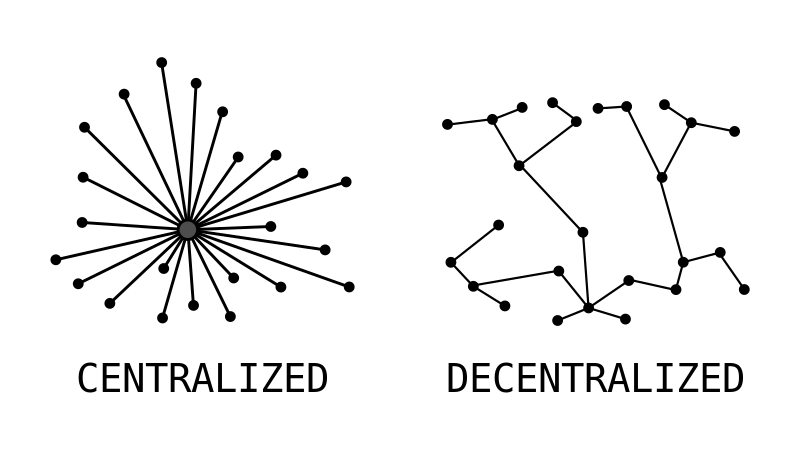
How can we create more trustworthy and accountable social media that support diverse communities? Decentralized social media—systems that allow users to connect and communicate across independent services like Mastodon or BlueSky—offer promising alternatives to centralized commercial platforms like Instagram, TikTok, or X. However, decentralized social media also face urgent design challenges, especially when it comes to content integrity, protecting community trust and safety, and forging collective governance. What happens when there is no central authority to review posts or ban abusive users? How can networks of autonomous communities build and adopt systems to govern effectively? What critical infrastructure can prevent the pervaisve harms of existing social media and support the integrity of public discourse?
Join Northwestern’s Center for Human-Computer Interaction + Design (HCI+D) and the Community Data Science Collective (CDSC) for an engaging conversation about the challenges and opportunites of decentralized social media on May 23rd from 4 to 5:15 p.m. CST. This panel features designers, leaders, and researchers involved in federated social media and will address opportunities for effective design and governance in this space.
Panelists include Jaz-Michael King, Bryan Newbold, and Christine Lemmer-Webber. Short presentations will be followed by discussion and Q&A moderated by Aaron Shaw (Northwestern HCI+D, CDSC).
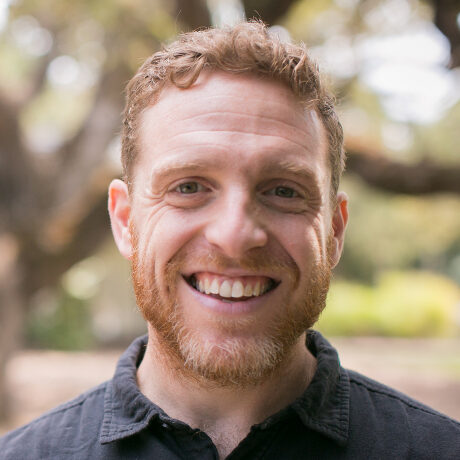
Aaron Shaw is Associate Professor of Communication Studies and Sociology (by courtesy) at Northwestern University and a Faculty Associate of the Berkman Klein Center for Internet and Society at Harvard University. He is a co-founder of the Community Data Science Collective. At Northwestern, he is also affiliated with the Center for Human-Computer Interaction + Design (HCI+D), the Institute for Policy Research, the Buffett Institute for Global Affairs, and the Public Affairs Residential College.

Christine has devoted her life to advancing user freedom. Realizing that the federated social web was fractured by a variety of incompatible protocols, she co-authored and shepherded ActivityPub‘s standardization. She has also contributed to many other free and open source projects, including co-founding MediaGoblin.
Christine established the open source Spritely Project to solve known problems in existing centralized and decentralized social media platforms and to re-imagine the way we build networked applications – work that now continues here at the institute under her guidance as Executive Director.
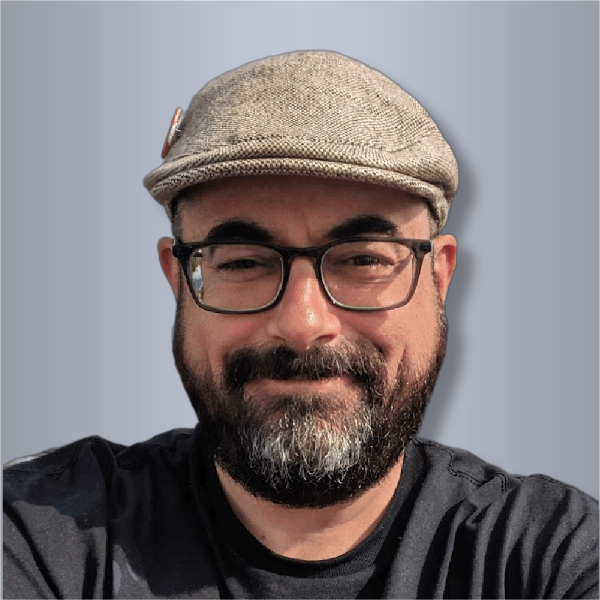
An accomplished professional with an extraordinary record of enabling data-driven decisions, developing innovative products, creating new business opportunities, driving strong operational performance, and building high-performing, agile teams.
Highly versatile, with extensive experience in data and technology from a privacy, improvement, and reporting perspective, Jaz has a proven record in building solutions for non-profit programs.
As Executive Director of IFTAS, Jaz is now focused on independent, open Social Web activities, with the aim of creating #BetterSocialMedia by supporting trust and safety at scale in federated social media networks.

Bryan works at Bluesky, a startup company building a federated social media protocol called “atproto”. Until a few months ago he worked at the Internet Archive collecting scientific research datasets and publications, and created scholar.archive.org. And before that he worked on infrastructure at Stripe, attended the Recurse Center in New York City, and built Atomic Magnetometers for a small New Jersey company called Twinleaf.
Over that same time period, Bryan climbed up and down the ladder of abstraction, obtaining an undergraduate degree in physics (at MIT), operating under-ice robots in Antarctica, developing open hardware lab instrumentation for large-scale brain probing (at LeafLabs), cataloging hundreds of millions of electronics components (at Octopart), and improved production service reliability at Stripe (a financial infrastructure start-up).
Bryan is a transplant from the East Coast and enjoys the road biking, large trees, generous salads, used bookstores, and world-class tech non-profits. This will be his third year serving on the Code of Conduct team at DWeb Camp.
Interested in attending? Register here to join!